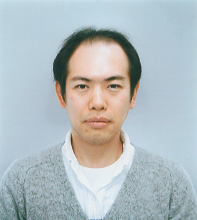
Hiroki Matsutani
Keio University, Japan
An On-Device Learning Approach for Unsupervised Anomaly Detection
Download SlidesAbstract
As an on-device learning approach, we will introduce a neural-network based online sequential learning and unsupervised anomaly detection (OSL-UAD) approach and its hardware design. The target domain is production lines in factories, but our application is currently expanding, such as anomaly detections in datacenter. One of the biggest issues when applying AI to industries is to prepare accurate training data sets before deployment, because noise pattern (e.g., vibration) fluctuates and status of products/tools varies with time. OSL-UAD learns normal patterns including noises in a deployed environment extemporarily to detect unusual ones, so no prior training is needed and well-suited for the on-device learning.
Biography
Hiroki Matsutani received the BA, ME, and PhD degrees from Keio University in 2004, 2006, and 2008, respectively. He is currently an associate professor in the Department of Information and Computer Science, Keio University. From 2009 to 2011, he was a research fellow in the Graduate School of Information Science and Technology, The University of Tokyo, and awarded a Research Fellowship of the Japan Society for the Promotion of Science (JSPS) for Young Scientists (SPD). His research interests include the areas of computer architecture, interconnection networks, and big data processing.