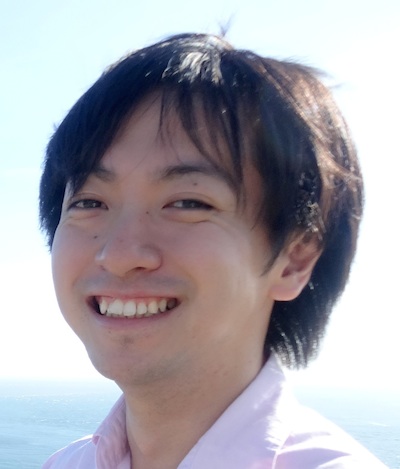
Shinya Takamaeda
Hokkaido University, Japan
QUEST: A Log-Quantized Deep Neural Network Engine with 3D Stacking SRAMs
Abstract
Deep neural network (DNN) is now fundamental machine learning technology in wide range applications. In use of edge computing, low-power inference accelerators of DNN are required for installing enough intelligence into embedded devices. In this presentation, we present a log-quantized neural network processor architecture and its LSI implementation. It employs hybrid datapathes of logarithmic region and linear region for eliminating the energy-consuming multiplier completely. The architecture supports variable bit-width of activation and weight values. Thus, computing performance and accuracy can be optimized to the application demands. In this talk, we introduce the design of our DNN processor and its evaluation results.
Biography
Shinya Takamaeda received the B.E, M.E, and D.E degrees from Tokyo Institute of Technology, Japan in 2009, 2011, and 2014 respectively. From 2011 to 2014, he was a JSPS research fellow (DC1). From 2014 to 2016, he was an assistant professor of Nara Institute of Science and Technology, Japan. Since 2016, he has been an associate professor of Hokkaido University, Japan. His research interests include FPGA computing, high level synthesis, and machine learning. He is a member of IEEE, IEICE, and IPSJ.