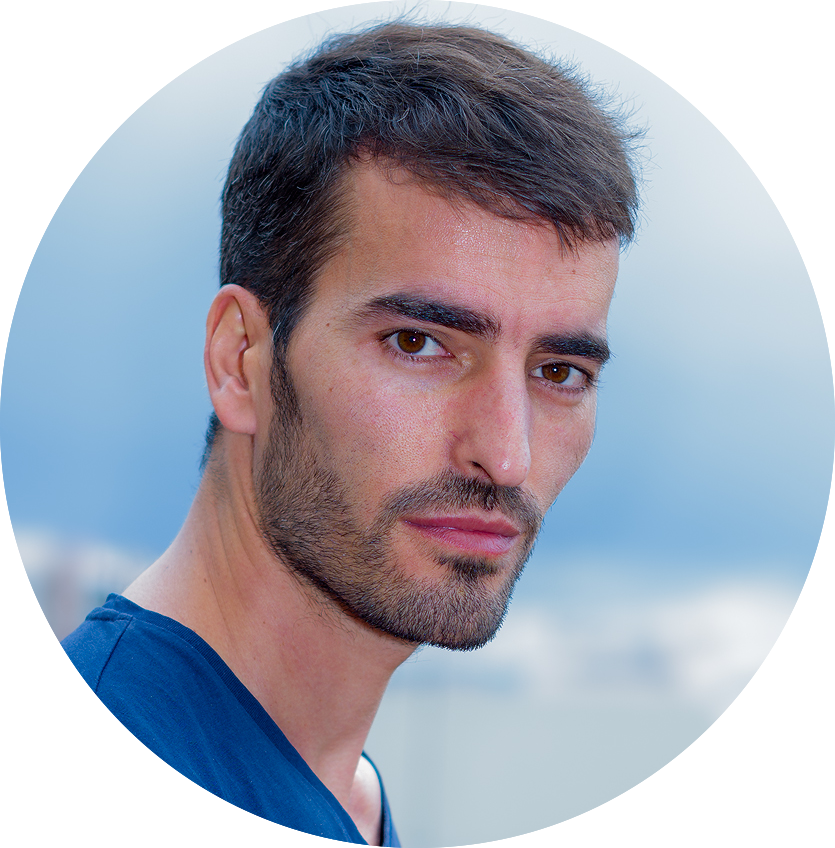
David Novo
LIRMM, Montpellier, France
Smart Data Movement across the Memory Hierarchy of Modern Computing Systems
Abstract
Data storage and movement is a fundamental bottleneck in modern computing systems, greatly limiting their overall performance and energy efficiency. This presentation introduces two new techniques to achieve smarter data movement across the memory hierarchy. The first technique uses perceptron-based prediction to identify off-chip load requests using multiple program features. When the load is predicted to go off-chip, it issues a speculative request directly to the memory controller. As a result, the on-chip cache access latency is removed from the critical path when the prediction is correct. The second technique uses reinforcement learning for data placement in hybrid storage systems. These systems use multiple different storage devices to provide high and scalable storage capacity at high performance. The new data placement technique observes different features of the running workload and the storage devices to make system-aware data placement decisions. Both techniques leverage machine learning algorithms to optimize data movement, significantly outperforming state-of-the-art solutions.
Biography
I am a CNRS research scientist (equivalent to assistant professor) in the ADAC Group at LIRMM, Montpellier, France, since January 2017. The LIRMM is a joint research unit owned by the French National Center for Scientific Research (CNRS) and the University of Montpellier (UM).
Previously, I was a post-doctoral researcher at the EPFL School of Computer and Communication Sciences, Switzerland, where I joined the Processor Architecture Laboratory (LAP) in November 2010. Even longer ago, I conducted my doctoral research at the Interuniversity Microelectronics Centre (imec), Belgium, receiving the Ph.D. in Engineering from the Katholieke Universiteit Leuven (KU Leuven) in 2010.
My research interests include hardware and software techniques for increasing computational efficiency in next-generation digital computers, with particular focus on memory systems for multi-core architectures and programmable accelerators for machine learning applications.
If you wish to modify any information or update your photo, please contact the Web Chair at the following address:
deep.samal[at]gmail.com