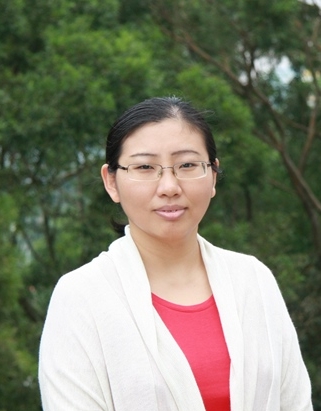
Wei Zhang
Hong Kong University of Science and Technology, Hong Kong
A History-based Auto-tuning Framework for Fast and High-performance DNN Design on GPU
Abstract
While Deep Neural Networks (DNNs) are becoming increasingly popular, there is a growing trend to accelerate the DNN applications on hardware platforms like GPUs, FPGAs, etc., to gain higher performance and efficiency. However, it is time-consuming to tune the performance for such platforms due to the large design space and the expensive cost to evaluate each design point. Although many tuning algorithms, such as XGBoost tuner and genetic algorithm (GA) tuner, have been proposed to guide the design space exploring process in the previous work, the timing issue still remains a critical problem. In this work, we propose a novel auto-tuning framework to optimize the DNN operator design on GPU by leveraging the tuning history efficiently in different scenarios. Our experiments show that we can achieve better performance than the state-of-the-art work, such as autotuning framework TVM and the handcraft optimized library cuDNN, while reducing the searching time by 8.96x and 4.58x comparing with XGBoost tuner and GA tuner in TVM.
Biography
Wei Zhang is currently an Associate Professor with the Department of Electronic and Computer Engineering, the Hong Kong University of Science and Technology, Hong Kong, where she established the Reconfigurable System Laboratory. She was an Assistant Professor with the School of Computer Engineering, Nanyang Technological University, Singapore, from 2010 to 2013. She authored over 100 technical papers in referred international journals and conferences and authored three book chapters. Her current research interests include reconfigurable system, hardware acceleration, power and energy management, and embedded system security. Her team has won the best paper award in ISVLSI 2009 and ICCAD 2017. She currently served in several editorial board, including IEEE TCAD, TVLSI, TRETS, ACM TECS and JETC.
If you wish to modify any information or update your photo, please contact the Web Chair at the following address:
deep.samal[at]gmail.com