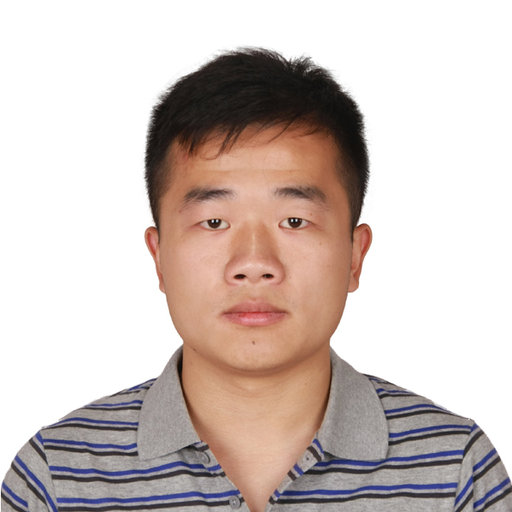
Xian Xiao
Hewlett Packard Labs
Large-Scale And Energy-Efficient Tensorized Optical Neural Networks
Abstract
Optical neural networks (ONNs) are expected to outperform electronic AI accelerators due to their ultra-low computational latency, high throughput, superior energy efficiency, and high parallelism. However, one major challenge for ONNs is the limited scalability. We propose a scalable and energy-efficient tensorized optical neural network (TONN) architecture on HPE’s densely integrated heterogeneous III-V-on-silicon device platform. The tensor-train decomposition enables the proposed architecture to be scalable to 1024 × 1024 and beyond. Furthermore, the footprint-energy efficiency ((MAC/J) · (MAC/s/mm^2)) of the TONNs can be improved by a factor of 1.4 × 10^4 compared with the state-of-the-art digital electronics.
Biography
Xian Xiao received the B.S. and M.S. degrees from Tsinghua University, Beijing, China, in 2012 and 2015, and a Ph.D. in electrical and computer engineering from the University of California, Davis, CA, USA, in 2021. He is currently a research scientist with Hewlett Packard Labs. Before joining Hewlett Packard Labs, he was a research intern with Nokia Bell Labs in the summer of 2016 and 2017, with Lawrence Berkeley National Laboratory from 2017 to 2018, and with Hewlett Packard Labs in the summer of 2018. He serves as a committee member of the OFC 2023 and PSC 2023 conferences. He has 50 conference and journal publications and holds 4 U.S. patents. His research interest includes neuromorphic computing, coherent Ising machine, III-V-on-silicon integration, and hybrid silicon comb laser.
If you wish to modify any information or update your photo, please contact the Web Chair at the following address:
deep.samal[at]gmail.com
Contact
Please address any issue to general chair Marilyn Wolf