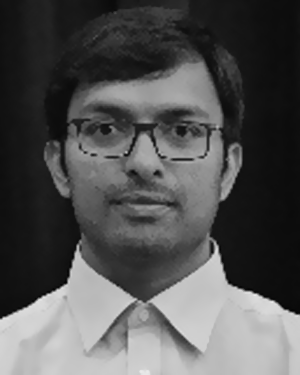
Yaswanth Raparti
AMD
Accelerating deep learning inference on client and edge devices
Abstract
Deep Learning models are deployed in a wide range of devices for smart applications such as vision, speech recognition, and recommendation. Particularly, with a growing focus on client, edge, and Internet-of-Things systems, the goal is to provide energy efficient inference capabilities while meeting the latency and throughput requirements of the user applications. This demands a combined effort in designing custom hardware accelerators and smart software optimization techniques. In this talk, I will give an overview of the AI accelerator solutions designed at AMD to address the challenges in DNN inference on client and edge devices to meet the performance and power constraints.
Biography
Yaswanth Raparti is currently a Manager – Systems Design Engineering, at Adaptive AI group in AMD. His area of research is HW/SW co-design of AI accelerators for high performance client and embedded processing systems. Prior to joining AMD, he worked at Micron, and Samsung in developing bleeding-edge memory solutions for cloud and data-center applications. He received his PhD in Electrical and Computer Engineering from Colorado State University in 2019, and B.E. in Electrical and Electronics Engineering from Birla Institute of Technology and Science, India.
If you wish to modify any information or update your photo, please contact the Web Chair at the following address:
deep.samal[at]gmail.com