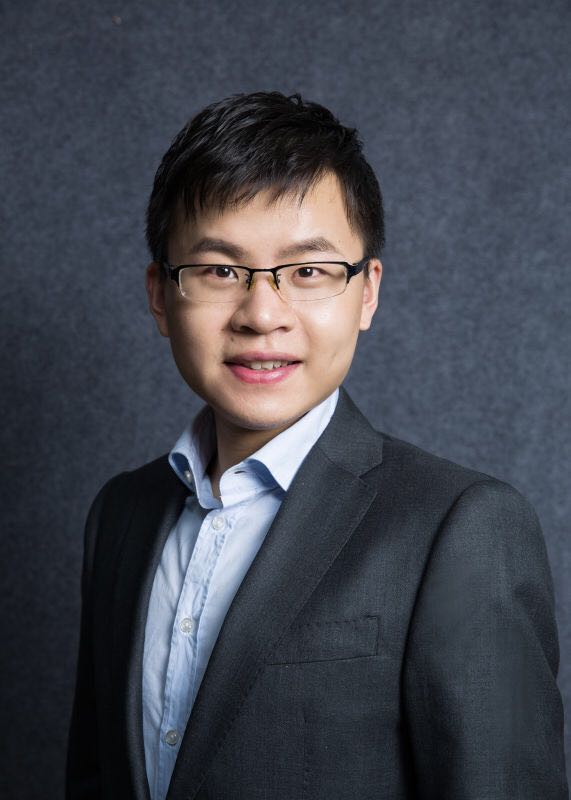
Song Yao
DeePhi Tech, China
The Evolution of Deep Learning Accelerators Upon Evolution of Deep Learning Algorithms
Abstract
The optimum architecture of deep learning processing units (DPUs) are coupled with applications and neural networks topologies. Though several classic neural networks such as AlexNet and VGG-Net are often used to evaluate the performance and efficiency of DPUs, they cannot reflect the real-world applications and deep learning algorithms have evolved far from those classic neural networks. First, new neural network topologies and functions popped up in the recent 2 years and have been widely used into practical algorithms. Besides, deep learning algorithms for real applications such as object detection and segmentation consist of not only a single neural network but also many other components. A practical DPU should consider both new neural networks topology and demands of real applications. In this talk, we will illustrate the evolution of DeePhi's Aristotle architecture for new neural networks and applications, together with the pre-silicon result for Tingtao ASIC. To make it easy to develop, a full-stack software tool called DNNDK (Deep Learning Development Toolkit) with tens of compilation optimization techniques is also proposed.
Biography
Song Yao received the B.S. degree in electronic engineering from Tsinghua University in 2015. His current research interests include model compression and deep learning acceleration. He is the founder and CEO of DeePhi Tech, an AI startup focuses on deep learning inference platform. So far DeePhi has received investment from Xilinx, Ant Financial, Samsung, MediaTek, and many top VCs. DeePhi's products for security, automotive, and data center have been widely adopted. DeePhi has been selected as top 10 AI startups in many charts. He is the recipient of Forbes 30 Under 30 Asia and MIT Tech Review 35 Under 35 Innovators award.