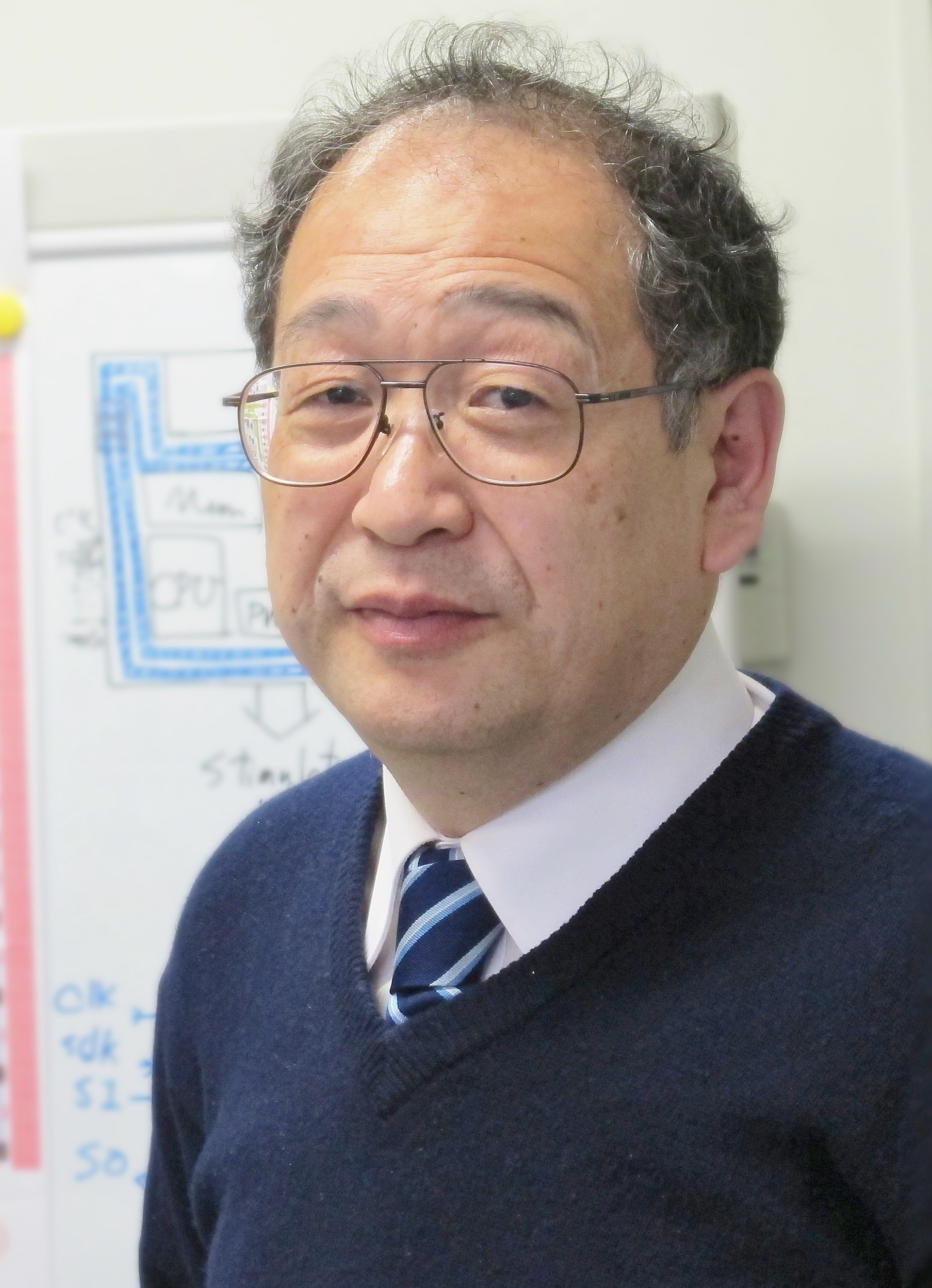
Yoshinori Takeuchi
Kindai University, Japan
Coding Analysis for Spiking Neural Network Models
Abstract
Spiking neural networks (SNNs) are neuromorphic computing models mimicking brain activity, which are expected to achieve high performance against artificial neural networks with low energy consumption. However, training SNNs with spike-timing-dependent plasticity requires intensive computational cost. Therefore, recent studies have focused the reduction of computational cost in training phase. In this presentation, we explain the analysis result for the multivariate trade-off between time resolution, training time, and performance of SNNs.
Biography
He is a Professor in the Department of Electric and Electronic Engineering at Kindai University. He received his B.E., M.E. and Dr. Eng. degrees from Tokyo Institute of Technology in 1987, 1989 and 1992, respectively. From 1996, he has been with the Osaka University. He was a visiting scholar in University of California, Irvine from 2006 to 2007. His research interests include System Level Design, VLSI design and VLSI CAD. He is a member of ACM, and Computer, CAS, SSC, and SP Society of IEEE.